- What Are Common Causes of .SVM Files Lost or Failure?
- How to recover lost .SVM files?
- Programs to recover .SVM files
- How to open file with .SVM extension?
What Are Common Causes of ".SVM" Files Lost or Failure?
There can be several common causes for ".SVM" file loss or failure. Some of them include:
- Accidental deletion: Users may accidentally delete ".SVM" files while trying to remove unnecessary files or folders.
- Software or hardware issues: Issues with the operating system, software conflicts, or hardware malfunctions can lead to file corruption or loss.
- Virus or malware attacks: Malicious software can infect and damage ".SVM" files, making them inaccessible or causing them to be deleted.
- Power failures: Abrupt power outages or system shutdowns can interrupt file saving processes, leading to file corruption or loss.
- Formatting or partitioning errors: Incorrect formatting or partitioning of storage devices can result in the loss of ".SVM" files.
- File system errors: File system errors, such as file system corruption or bad sectors on the storage device, can cause ".SVM" file failure or loss.
- Human error: Mistakes made by users, such as accidental formatting of storage devices or improper handling of files, can lead to ".SVM" file loss.
- Software bugs or glitches: Bugs or glitches in software applications that handle ".SVM" files can cause data corruption or loss.
- Physical damage to storage devices: Physical damage to hard drives, USB drives, or other storage devices can result in the loss of ".SVM" files.
- Synchronization or backup failures: Failures during the synchronization or backup process can lead to ".SVM" file loss if the files were not properly backed up.
It is always recommended to regularly backup important files and use reliable antivirus software to minimize the risk of ".SVM" file loss or failure.
How to recover lost ".SVM" files?
Sometimes while working with a computer, laptop or other stationary or mobile devices, you may encounter various bugs, freezes, hardware or software failures, even in spite of regular updates and junk cleaning. As a result, an important ".SVM" file may be deleted.
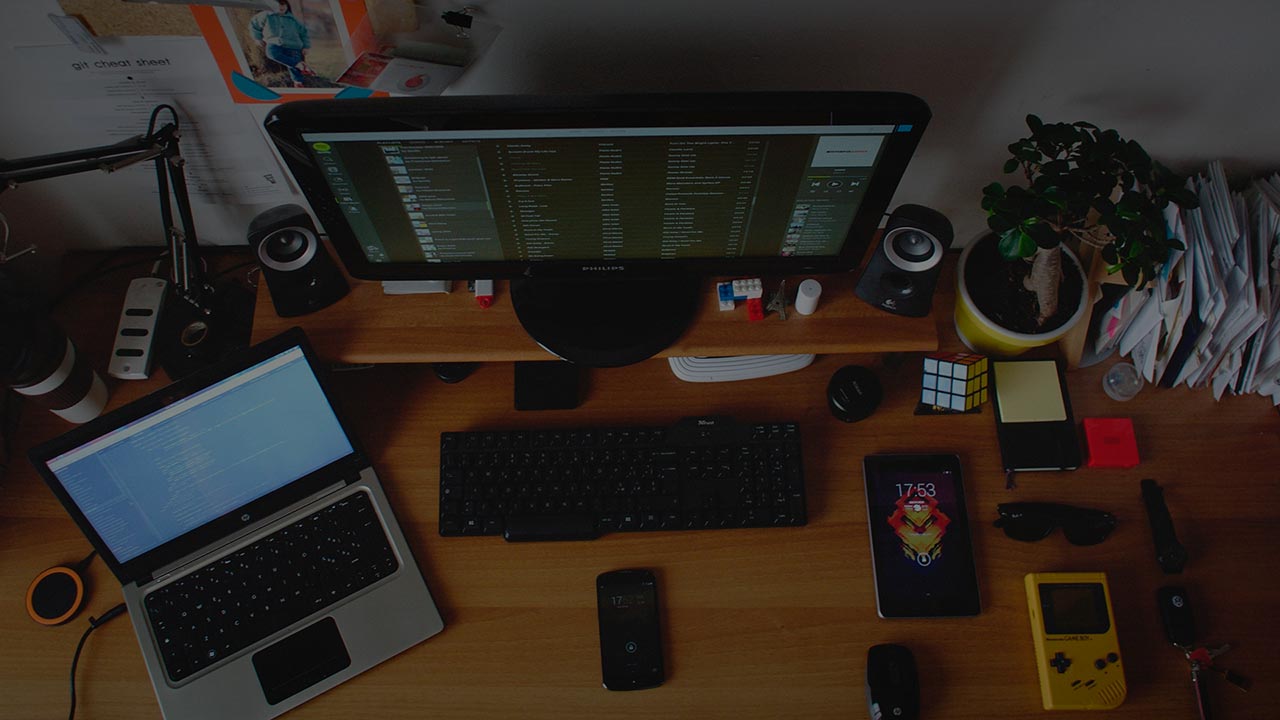
🧺 How to Recover Files and Folders After Sending Them to the Recycle Bin and Deleting? (Windows 11)
By no means should you think that the only way to recover a ".SVM" file is always to create it once more.
Use programs for recovering ".SVM" files if a file was lost after accidental or deliberate deleting, formatting the memory card or the internal storage, cleaning the storage device, after a virus attack or a system failure.
Programs to recover ".SVM" files
Looking for a way to get files back? In cases when files were deleted and they cannot be restored by using standard operating system tools, use Hetman Partition Recovery.
Follow the directions below:
-
Download Hetman Partition Recovery, install and start the program.
-
The program will automatically scan the computer and display all hard disks and removable drives connected to it, as well as physical and local disks.
-
Double-click on the disk from which you need to recover ".SVM" files, and select analysis type.
-
When the scanning is over, you will be shown the files for recovery.
-
To find a file you need, use the program’s interface to open the folder it was deleted from, or go to the folder "Content-Aware Analysis" and select the required file type.
-
Select the files you have been looking for and click "Recovery".
-
Choose one of the methods for saving the files and recover them.
How to open file with ".SVM" extension?
Looking for how to open a stereo starView Metafile image file file?
Programs that open ".SVM" files
Windows |
---|
![]() |
![]() |
Mac |
![]() |
![]() |
Linux |
![]() |
![]() |
Additional Information
-
File type: StarView Metafile
-
File extension: .SVM
-
Developer: StarDivision
-
Category: Raster Image Files
-
Format: N/A